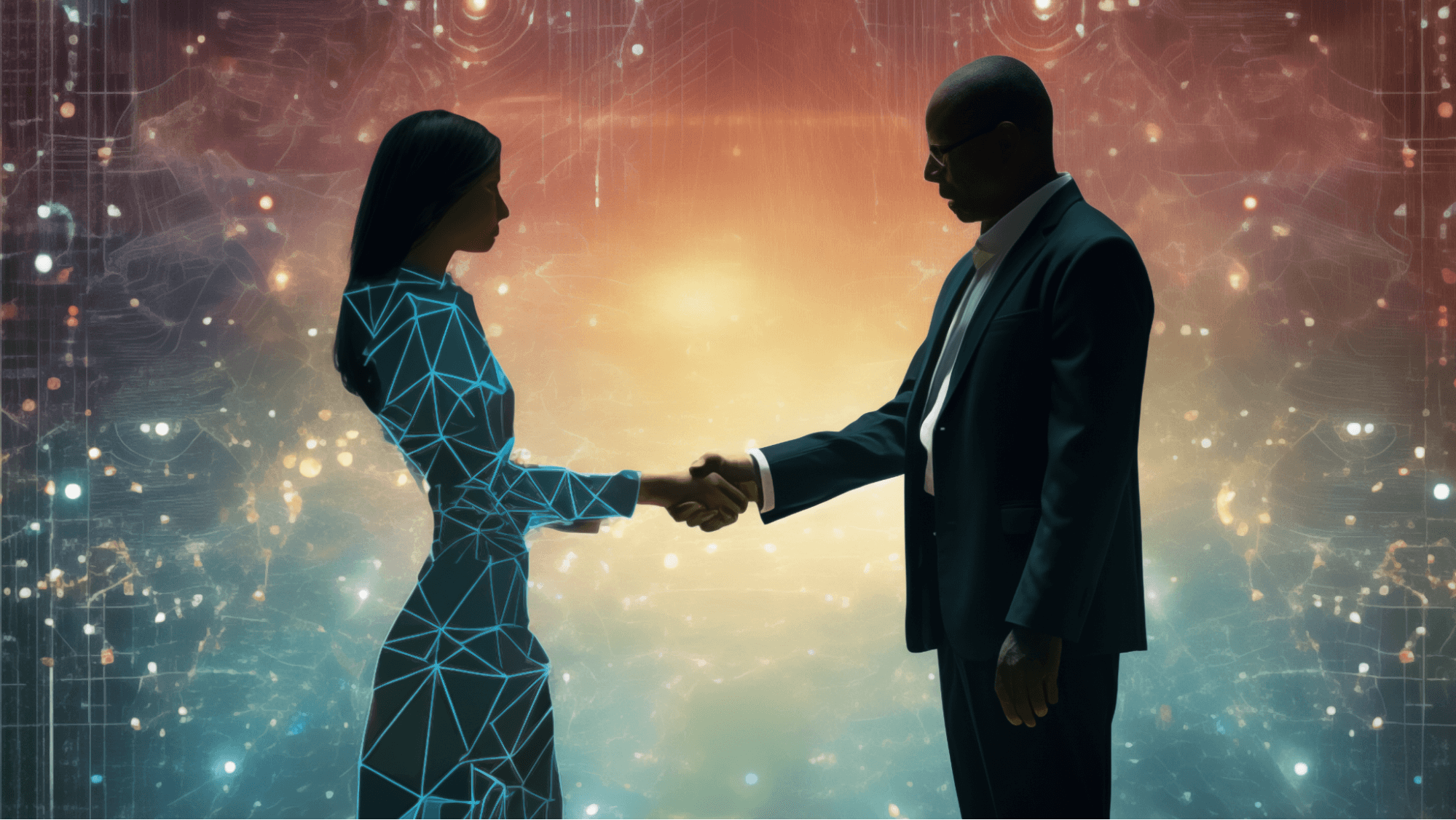
There's a new symbiotic relationship emerging in tech: AI startups need data to compete with incumbents, and corporations need AI innovation to compete with each other. But how can they build relationships to safely and profitably collaborate? It's all about building trust—in each other's security, longevity, and desire for a win-win outcome.
To lay out a playbook for building AI data partnerships, we brought together top tech leaders and startup lawyers for a SignalFire AI Lab Live event at our SF headquarters. They shared tips for how to initiate, negotiate, and carry out data deals. The key takeaways included:
- It's not always about being the smartest startup that makes a pitch: be the most empathetic one that understands your corporate partner’s needs and fears.
- AI startups should focus on solving less sensitive internal problems for corporations as a way to build trust and unlock access to bigger, more sensitive data sets.
- Enterprises are increasingly scrutinizing their data rights when forming partnerships. They want the ability to extract their data from a startup's AI model, control how and in what markets it's applied, and ensure continuity if the startup fails.
- Beyond data, corporate partners can offer startups a robust testing environment, critical product feedback, and the halo effect that comes from a well-known corporate logo becoming an initial customer.
- How AI regulation shakes out will depend on several key data and copyright court cases this year. In the meantime, startups can minimize risk for customers by using synthetic data, offering legal coverage, and closely tracking their data sources.
![]() |
In this blog, we share all the top insights on AI data licensing from our event, featuring:
- Training data provider Scale AI’s Field CTO Vijay Karunamurthy
- Legal software startup Everlaw’s Chief Legal Officer Shana Simmons
- Healthcare AI startup CodaMetrix’s CEO Hamid Tabatabaie
- Law firm Goodwin Proctor’s Partner Kevin Lam
- Our venture firm SignalFire's AI Lab lead and Principal Veronica Mercado
If you're building something special in AI and want access to help with compute, recruiting, marketing, and data partnerships, SignalFire would be excited to chat with you!
Building trust with AI customers
"When you are a founder selling AI, you're really selling a trajectory," Karunamurthy laid out as we started the panel. Unlike traditional, more stable software that's designed to solve a current problem, AI is evolving so quickly that you need to convince buyers that your future product will solve their future problems. They need to believe in your roadmap, your team, and your vision for how the world will change.
![]() |
That requires finding an internal champion and understanding their own motivations. Do they care about cutting costs, unlocking new capabilities, or even just building a reputation of innovation for their company? For example, health systems competing for patients, doctors, and partnerships are constantly looking for ways to differentiate from their peers. They need to have the deep conviction to keep advocating for you if your initial projects don't pan out and you need time to build what you promised.
But to earn the chance to land-and-expand and deliver on your vision, it's easiest to first solve an internal problem with lower stakes. "If you're one of 100 founders in front of a company trying to get data, how do you separate yourself from the 99?” asks Lam. “You have to create a bridge of trust. The way to do that is to start slowly, kind of open the door a little bit at a time."
![]() |
Especially in regulated industries like healthcare with strict rules about privacy, working with external customer data can be risky. "In business, the two things you can get wrong for someone is their money—so their billing—and their data, especially the stuff that comes up in investigations [like email]," Everlaw’s Simmons said about where to be careful. But if you can earn their trust, your partnership will expand, they can become a robust testing environment for your product, and they can become a powerful reference that engenders faith from future customers.
Types of Data Partnerships: Licensing, sharing, and consortiums
How do AI data partnerships work? The two common forms are data licensing agreements where one party pays for access to the other’s data, and data sharing deals where a business provides data access alongside payment for another’s product.
Data licensing agreements can function like the one OpenAI signed with the Associated Press to train the tech giant's models on the AP's journalism. Customer relationships look more like data in exchange for discounts and/or the promise of improved service.
Additionally, some companies create a data consortium, like SignalFire portfolio company CodaMetrix, which uses AI for medical bill coding. Consortium members—CodaMetrix’s customers—opt-in to contribute their data for use by fellow members in exchange for a revenue share.
![]() |
Data governance questions that every AI startup needs to answer
When you partner up, you assume responsibility for your partners' data. That means verifying your own security, privacy, and governance policies, as well as those of any technology vendor you integrate with, to keep partners' data safe.
Savvy corporate partners will scrutinize your data agreements and safeguards, so some questions you should have answered ahead of time include:
- Are there specific limits on how the data can be applied, such as for training your model or fixing bugs?
- Can the partner's data be siloed or even excised from AI models if necessary?
- Are roles, access permissions, and data lineage tightly managed and auditable?
- Are you able to navigate legal nuances and regulations in different geographies?
- Is any personally identifiable information omitted or at least anonymized and aggregated?
- Can you prove this sensitive data can't be reverse-engineered or de-anonymized later?
- Do you guarantee not to use the data to compete with the partner in the future?
- Was your training data legally collected, and is there any legal risk for the customer?
- If your startup fails, will the customer still have access to the product their data helped build?
Unfortunately, providing these assurances can take a ton of work. Preemptively attaining certifications like FedRAMP (Federal Risk and Authorization Management Program) can boost confidence in your practices, especially if you're working with regulated customers.
That’s why throughout the process, cooperative communication is key. Most startups can’t afford to fight a court case against a large customer who copies their product. Removing the influence of a customer’s data on a startup’s model is costly and labor intensive, like trying to “unscramble an egg,” as CodaMetrix’s Tabatabaie said. It’s much better to disarm those problems before they emerge.
![]() |
Navigating the legal uncertainty of AI
The fate of many AI model training schemes and business models hinges on the outcomes of several high-profile court cases this year. Most center on whether AI models illegally used artists, authors, musicians, and programmers' work as training data without their consent. Decisions favoring the plaintiffs could open up even more lawsuits against developers and force them to secure licensing deals going forward that could drag down profitability.
Here is a list of some of the major lawsuits against AI companies as of late January 2024:
- GitHub users are suing its owner Microsoft over using their code to train its GitHub Copilot programming assistant, though some of the plaintiffs’ claims have been dismissed.
- Artists are suing image generation tools, including Midjourney, Stability AI, and DeviantArt over training their models on these artists’ work without consent.
- Stock image giant Getty is suing Stability AI over using its trove of photos and illustrations to train its model, evidenced by Getty watermarks showing in images produced with Stability.
- The New York Times is suing OpenAI over scraping its archive of articles to train ChatGPT, which the publication claims repeats its content verbatim and mimics its writing style.
- Book authors are suing EleutherAI as well as OpenAI and Meta for allegedly scraping their copyrighted works to compile EleutherAI’s dataset, “The Pile,” which has been licensed to train these tech giants’ AI models.
- Universal Music Group is suing Anthropic for scraping lyrics to copyrighted music to train its chatbot.
Until there’s more legal precedent, startups should be mindful of where they’re sourcing training data as well as how directly that data can show up in outputs. To reassure customers, some AI companies are even pledging to pay for legal costs if any of their customers are sued for using their products.
With the need for trust in AI’s utility, data governance, and legality, corporate partners may benefit from working through a credible intermediary that can help them find reliable partners. And given the understandable concerns of buyers, startups with allies that can make introductions and vouch for their data standards will have the advantage. That’s why SignalFire established its AI Lab, which serves as a bridge between corporates that have problems but plenty of data, and founders that have solutions they want to refine. We provide the next category-defining AI startups with partnership introductions, talent, capital, and other resources they need to build better and faster.
The companies, large and small, that are willing to wade through today’s uncertainty will be the ones that create the iconic products of tomorrow. There’s no shortage of opportunities across enterprise, healthcare, security, developer tooling, and more that can finally be addressed affordably through AI’s scale. But the answers won’t be built alone, and their success will hinge on our willingness to dream and collaborate together, which makes us human.
*Portfolio company founders listed above have not received any compensation for this feedback and did not invest in a SignalFire fund. Please refer to our disclosures page for additional disclosures.
Related posts
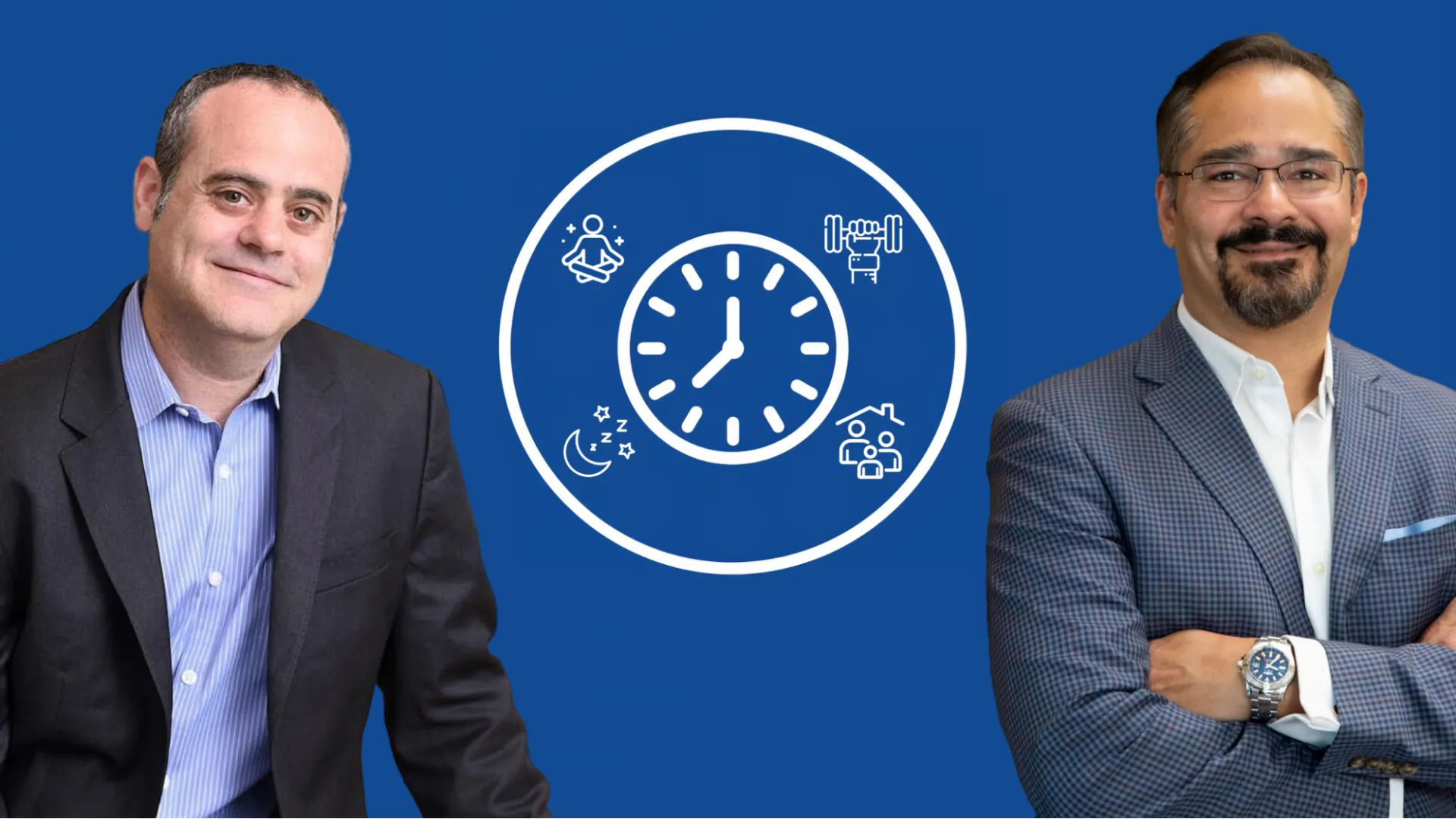