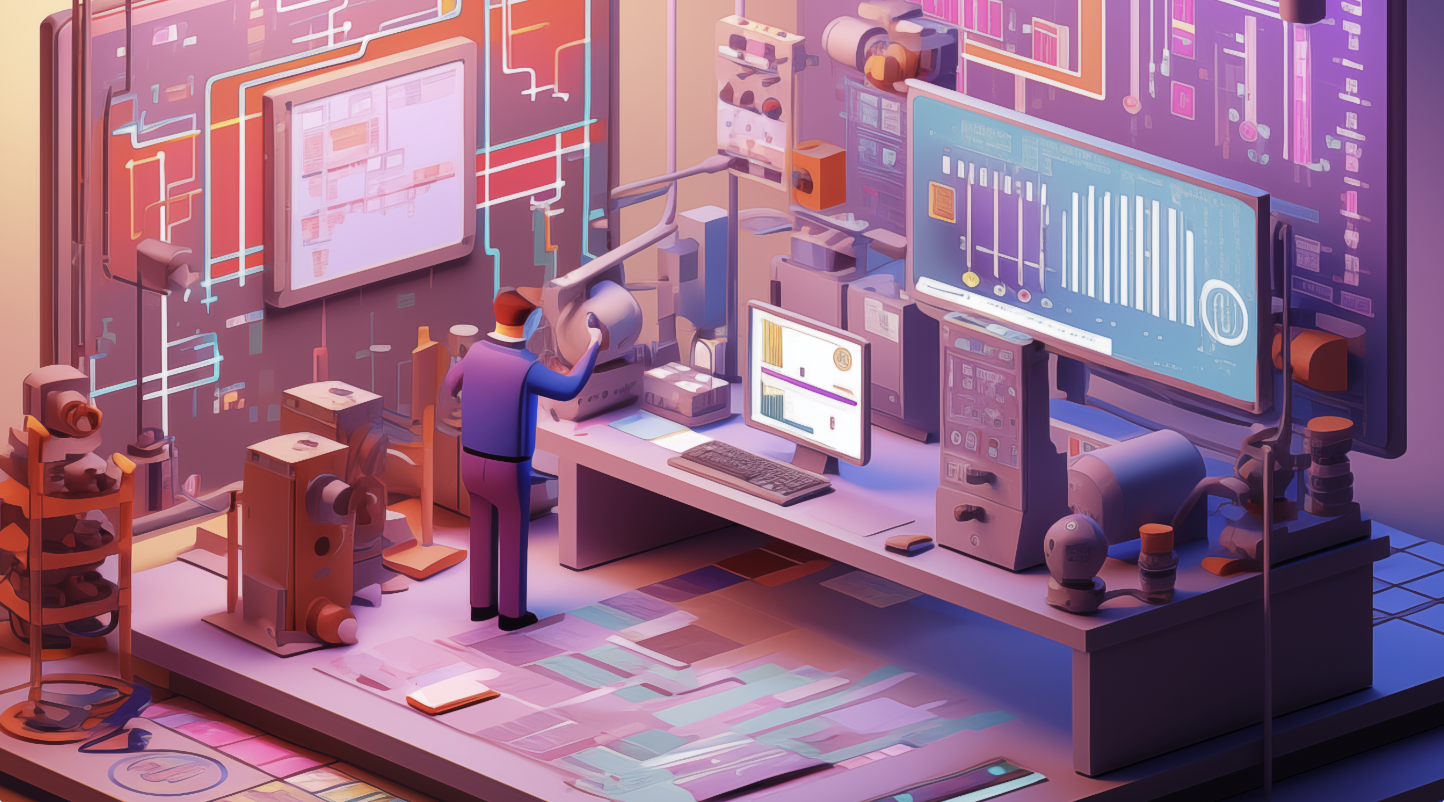
Data volumes are exploding—in the time it took you to read this sentence, over 25,000 terabytes of data were created around the world. And by 2025, estimates indicate 80% of that data will reside in large enterprise environments. The problem is that much of that enterprise data suffers from significant quality issues as it gets put to use.
And this is important—enterprises say data quality issues are the number one bottleneck for further enterprise AI adoption.
Modern enterprises rely on high-quality data to run their businesses, in the same way that automotive manufacturers rely on high-quality parts to produce reliable cars. First, the raw materials are collected (data pipelines, in our world.) Second, they are brought onto the factory floor (loaded into a data warehouse; e.g., DataBricks, Snowflake, BigQuery). Next, within the data warehouse, the raw materials (data) are combined and manipulated, step-by-step, much like an assembly line. At the end of the process is the finished good—whether it’s a customer-facing data product or insights/recommendations for management on how to run the business.
![]() |
The integrity of the data value chain is critical here: with incorrect inputs or transformations, even large, sophisticated enterprises can make erroneous business decisions, sign suboptimal MSAs, misprice their offerings, ship faulty products, or disappoint customers.
Going back to the factory, how do we know that defects aren’t being introduced at each step of the assembly line? And if the product does come out defective, how can we most quickly find the root cause, correct the issue and get the factory up and running properly again?
Anomalo can help. Today, we’re announcing that SignalFire has just led Anomalo’s $33M Series B to help it solve the data quality problem for enterprises around the world. You can read more about the news in TechCrunch.
Using AI, they identify the root cause of data quality issues at enterprise scale. Whether it’s a broken data pipeline, stale or outdated data, individual anomalous values skewing distributions, unintended mix shift, seasonality, or another confounding variable, Anomalo will find it, explain it to data owners, and allow them to solve real business problems.
Businesses spend over $66 billion annually on data infrastructure, but that spending is pointless if the underlying data is jumbled or erroneous. In the age of AI, enterprises are investing in modernizing their data environments—and now Anomalo lets them monitor and address their data quality.
Anomalo’s AI unlocks new capabilities for data quality
Complex data environments have long been plagued with problems, but Anomalo is ready to solve them. Three things have changed to create the “why now” for Anomalo:
- As mentioned above, there’s exponentially more data and thus exponentially more complexity in data warehouses.
- Data warehouses themselves have matured significantly and gained meaningful enterprise adoption, creating a starting point for quality analysis.
- Modern technology like machine learning has made it possible to solve the data quality problem much more efficiently.
![]() |
Why exactly is machine learning necessary? Going back to the early days of data hygiene, if you were trying to identify issues in your data, you might start with something simple:
Rules-Based Data Hygiene (Gen 1): For example, write a rule that anytime (Price * Volume) does not equal GMV for a given transaction, flag an anomaly. This can be done easily in SQL. It has the advantage of being well defined, but the large disadvantage is that there are near-infinite rules that need to be followed in enterprise-scale datasets. Additionally, rules can change over time and have exceptions, so scripting thousands of hard-and-fast rules creates alert fatigue, which has been the downfall of oh-so-many companies in both the IT and cybersecurity spaces. In short, data teams simply ignore the anomalies being flagged because of too many false positives.
Metrics-Based Data Observability (Gen 2): Okay, so a rules-based approach doesn’t scale. What if you take all your tabular data, add baseline metrics (number of entries per day, average value, etc.), and screen for outliers? Well, this is certainly an improvement: it allows for more dynamic rules and can scale to larger environments. But fundamentally, it’s based on metadata—that is, information about the data table itself—things like lineage, freshness, and summary variables. Unfortunately, this approach has two problems. First, it doesn’t give you any explanatory power. An alert that says, “Hey, today’s table has too few entries” leads to a frustrating investigation process rather than a quick remediation. Second, it doesn’t catch nuanced anomalies. Slow drifts in the types of data coming in, mix shift between categories, seasonality, and more—these all require more than just metadata analysis to analyze.
Enter Anomalo’s differentiating feature, which is:
AI-Based Data Quality Platform (Gen 3+): Anomalo’s unsupervised machine learning approach analyzes data at the record level—it can identify individual data points or groups of data points that are indicative of the problem in the system and flag them to the data team. Critically, Anomalo’s platform is the only one in the market with powerful root cause analysis: data teams don’t just see, “Hey, something’s probably wrong in this data table,” but rather, “Hey, this specific data point here is off. It seems to be due to a change in the data coming from this particular data source.” This wasn’t possible until recent advances in machine learning, and Anomalo’s team is composed of engineers and data scientists that have been right at the heart of those innovations.
Assembling the all-star team for data quality
Few companies deal with data quality at scale like grocery deliverer Instacart, where Anomalo’s co-founders Elliot Shmukler (CEO) and Jeremy Stanley (CTO) met. Elliot was chief growth officer and Jeremy was VP of data science. Elliot’s team was heavily data-driven, using data signals to identify where to launch Instacart’s next market, how to optimize the product for growth, and how to target users with promotions and offers. He saw firsthand what can happen if data pipelines fail or anomalies are left unaddressed—business outcomes are directly impacted.
As part of our investment, they’ll now be supported by SignalFire executive-in-residence (XIR) Doug Merritt*, the former CEO of Splunk (recently acquired by Cisco for $28 billion) and current CEO of Aviatrix (a network security business valued at $2 billion). He’s intimately familiar with the enterprise data environment, having led a team that helped keep Fortune 500 data environments secure at scale. Doug is investing alongside SignalFire and joining Anomalo as a strategic board advisor. We’ve been working with Doug as part of our XIR program for the past six months to find a data infrastructure company with a leadership team capable of solving the enterprise’s hardest problems today. We first met Anomalo in November, and after speaking with a number of customers, competitors, and the management team, knew we’d found the winner.
Through my decades of experience in the big data industry, including my tenure as CEO of Splunk, I heard from countless customers who were struggling to find a solution to their data observability and quality issues. They tried everything from manual data cleansing to implementing complex data governance programs, but nothing fully addressed the problem. That was until I learned about Anomalo.
Anomalo's approach to data observability and quality is unlike anything I've seen before. Their cutting-edge technology and talented team have created a solution that is not only effective but also easy to use. The Anomalo team is uniquely talented, and their passion for data quality is evident in everything they do. I'm excited to see how Anomalo continues to help organizations of all sizes unlock the full potential of their data.
—Doug Merritt, SignalFire XIR*, former CEO of Splunk
We are lucky enough to have Databricks, a leading data warehouse, co-investing in the round alongside us. Additionally, we’re joined by a fantastic slate of existing investors: Norwest Venture Partners, Foundation Capital, and Two Sigma Ventures.
Customers tell the story
We commonly hear complaints from customers when we perform due diligence for enterprise tools—things like long implementation cycles, overpromised features, and other small nitpicks. Here, the story was different. Anomalo’s customers have simply glowing reviews for its product, with one Fortune 500 VP of data science telling us they were “the best technology partner we’ve ever had for anything, period.”
And they’re speaking with their dollars, not just their words. The company ended fiscal Q3 with 2.8x year-over-year growth and doubled their number of Fortune 500 customers.
![]() |
We understand those customers because we share their need for data quality. SignalFire is an AI-native firm that takes a data-driven approach to investing and portfolio support. We’ve spent the last decade building our own Beacon AI data platform, which crunches more than a half-trillion data points to rank over 600 million people and companies in the global tech ecosystem on quality and hire-ability. It helps us identify incredible technical talent, fast-growing companies, active open-source projects, and more, while also powering an elite talent search engine and customer-lead lists for our portfolio companies.
We were actually the very first customer of another data observability company going back 5+ years, and have been on the lookout for a modern, AI-equipped solution to our problems. keenly aware of the need for this solution. Beacon surfaced that the quality of Anomalo’s engineering and business talent was off the charts.
Anomalo’s CEO Elliot Shmukler shared why this sector expertise, and SignalFire’s unique approach (both our core platform and the XIR program), resonated with him when we first met:
![]() |
With a modern approach to a massive problem, a beloved product built by an expert team, and now with the support of SignalFire XIR Doug Merritt’s experienced leadership, Anomalo is primed for exceptional growth and we are humbled to be part of the journey.
* Disclosure: SignalFire may engage Affiliate Advisors, Retained Advisors, and other consultants as listed above to provide their expertise on a formal or ad hoc basis. They are not employed by SignalFire and do not provide investment advisory services to clients on behalf of SignalFire. For more information on their specific roles, please contact us. Certain portfolio company founders listed on our website have not received any compensation for this feedback and did not invest in a SignalFire fund.
*Portfolio company founders listed above have not received any compensation for this feedback and did not invest in a SignalFire fund. Please refer to our disclosures page for additional disclosures.
Subscribe to our newsletter
By submitting this form, you agree to receive marketing communications from SignalFire.See our privacy policy for more.
Share
Related posts
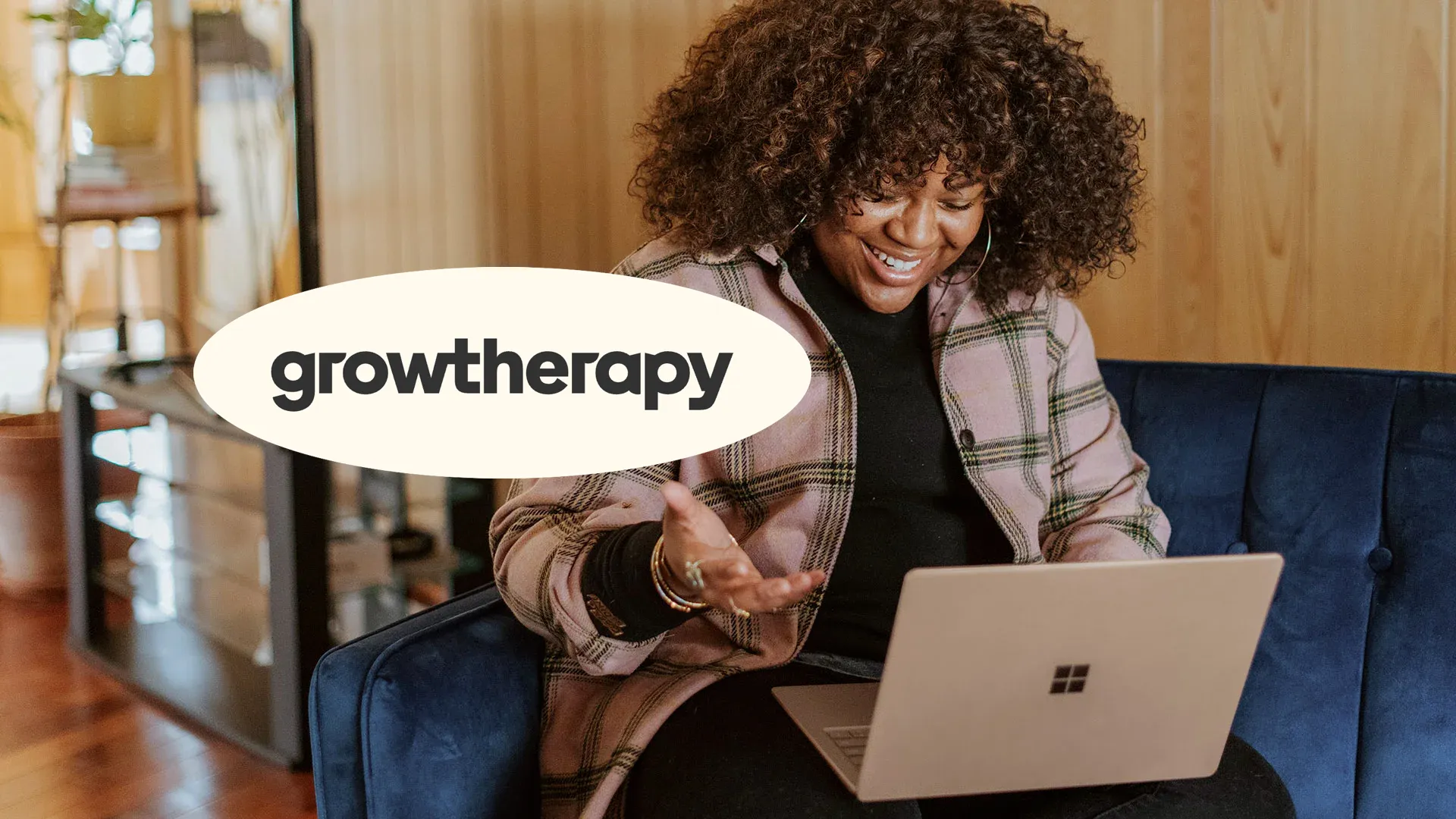